- ExoBrain weekly AI news
- Posts
- ExoBrain weekly AI news
ExoBrain weekly AI news
21st March 2025: The Superbowl of AI, Adobe orchestrates autonomous marketing, and is agent capability doubling every seven months?
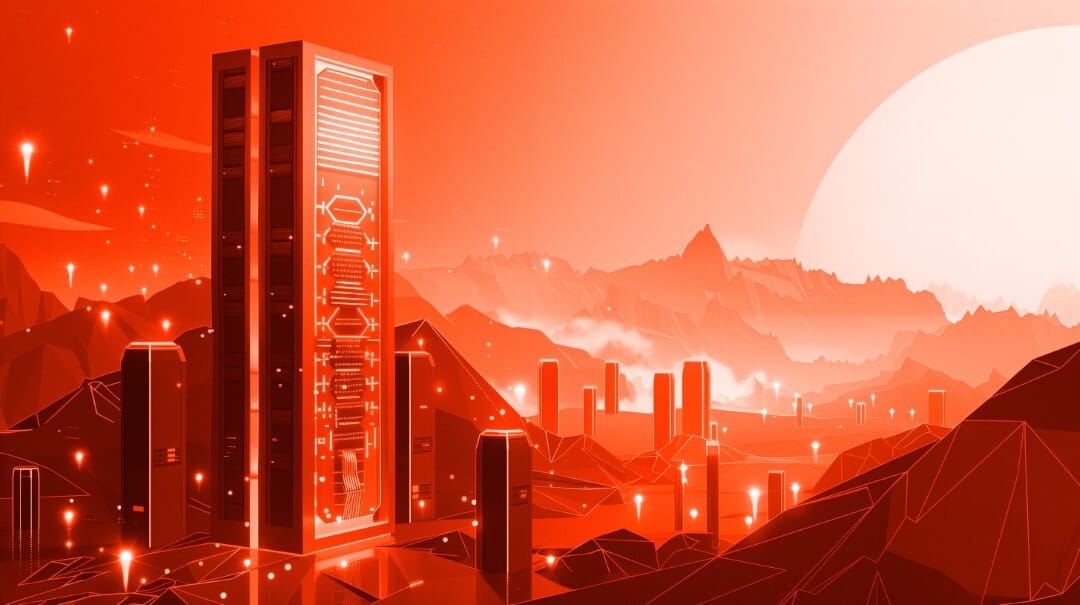
Welcome to our weekly email newsletter, a combination of thematic insights from the founders at ExoBrain, and a broader news roundup from our AI platform Exo…
Themes this week:
Nvidia's GTC 2025 event and hardware roadmap through 2027
Adobe's push into marketing AI with 10 new agents
Research suggesting AI capabilities are experiencing a new Moore's law
The Superbowl of AI
When Nvidia held its first developer conference in 2009, it was a modest gathering in a hotel with academics standing by poster boards. This year, GTC 2025 saw more than 25,000 attendees fill an NHL arena, with San Jose decked out in Nvidia's neon green and black. The event has transformed from an academic gathering to a self-styled "Superbowl of AI". At this week's extravaganza, Nvidia unveiled its next-generation AI chips and laid out a relentless three-year roadmap demonstrating every confidence that massive AI infrastructure spending will continue. Despite these announcements, Nvidia's shares slipped, highlighting the market's uncertainty about the geopolitical landscape, tariffs and long-term demand.
In a lengthy and detailed keynote delivered without notes or autocue, CEO Jensen Huang announced that Blackwell Ultra, the successor to Nvidia's current flagship AI chip, that will ship in the second half of 2025. While Nvidia's stock has dropped this year, this reflects broader market risk sentiment rather than a genuine threat to its business model from the likes of DeepSeek. In fact, more efficient models are likely to expand AI usage, not shrink it, just as better video compression didn't reduce streaming but enabled more content on more devices. "The amount of computation we need at this point as a result of agentic AI, as a result of reasoning, is easily 100 times more than we thought we needed," Huang told the GTC audience. This statement reveals Nvidia's strategy: betting that AI's future lies not just in efficiency but in massive scale.
Huang outlined the next wave of AI development, “agentic AI” with a further wave, “physical AI” for robotics and self-driving cars, already emerging. The shift to agentic AI dominated the conference. Nvidia unveiled a new family of models with advanced reasoning capabilities, specifically designed to power autonomous agents, and AI-Q Blueprint, a framework for connecting knowledge bases to AI agents that can act autonomously. This represents Nvidia's vision of AI systems that can reason through problems, make decisions, and take actions with minimal human oversight. To support this vision, Nvidia launched Dynamo, an open-source software framework that splits AI reasoning across multiple GPUs. By disaggregating workloads, Dynamo can double AI performance and boost token throughput by 30 times when running models like DeepSeek-R1.
Nvidia also announced new DGX “personal supercomputers”. These highly compact systems pack cut-down Blackwell GB10 GPUs into PC form factors. They’re available for pre-order but aren’t cheap, starting at an eye watering $2,999. Others are getting into the AI desktop business, with Dell unveiling its Pro Max GB300 workstation, a behemoth capable of delivering a staggering 20 petaFLOPS. Dell is also preparing a mini workstation based on the GB10 processor. Meanwhile, HPE and Lenovo are readying their own solutions, with HPE's ProLiant servers supporting various Blackwell configurations.
At the opposite end of the spectrum, Nvidia unveiled the astonishing NVL576 "Kyber” rack system. This liquid-cooled thing of beauty will house 576 GPUs in a single rack and will consume perhaps as much as 600 kW of power when it ships in the second half of 2027. For context, three years ago, Meta's entire AI research datacentre (then the world's most powerful) delivered similar performance. What once required a building now fits in a single rack.
The power density figures are mind-boggling. Current Blackwell B200 racks consume around 120 kW, but the Kyber infrastructure will quintuple that figure. Each Kyber rack will consist of four 'pods', with each pod housing 18 blades, and each blade supporting eight Rubin Ultra GPUs. That's 144 GPUs per pod, designed to deliver up to 15 exaflops of FP4 inference performance. This extreme density creates new challenges for power and cooling, with Nvidia developing specialised liquid cooling technologies to manage the heat output. Looking even further ahead, Huang suggested that future datacentre racks in Nvidia’s “AI factories” might require megawatts of power each, an unprecedented concentration of computing power that will demand entirely new approaches to design, power delivery, and thermal management.
One of the most interesting keynote slides was a graph comparing Hopper to Blackwell. The graph plotted throughput (tokens per second per megawatt) against responsiveness (tokens per second per user), revealing Blackwell's ability to process 25 times more data than Hopper while maintaining the same energy consumption. With each generation of Nvidia chips, the performance-per-watt curve shifts dramatically upward, enabling entirely new classes of AI applications, and it’s this fundamental equation that’s unlocking the agent revolution. Consider what this means in practical terms: a unit of compute that could run 1,000 concurrent AI agents using Hopper can now run 25,000 with Blackwell, and many multiples more with future Rubin Ultra chips, all while consuming the same amount of power. This doesn't just improve existing applications; it enables completely new ones that weren't economically viable before.
GTC wasn't just about chips and infrastructure. Nvidia showcased significant advancements in robotics through the Isaac GR00T N1 project in partnership with Walt Disney and Google DeepMind, aimed at accelerating the development of robots with advanced manipulation abilities. The automotive sector saw major announcements too, with Nvidia partnering with General Motors to develop next-generation automotive AI systems. On the networking front, collaborations with T-Mobile and Cisco will develop AI-powered 6G hardware. Healthcare applications featured prominently, with new AI tools for drug discovery and medical imaging. The breadth of industrial applications, from digital twin simulations for manufacturing to computer vision systems for quality control, highlighted how Nvidia is trying to drive demand for its hardware by envisioning application in every sector.
Quantum also made an appearance at the event, despite Huang previously seeking to keep the industry focused on Nvidia’s “classical” accelerated computing. The quantum computing efforts at GTC suggest that Nvidia is now positioning itself for multiple futures. Quantum and classical computing will likely coexist and complement each other, with quantum taking some workloads while also generating new classical demand.
Takeaways: Nvidia's “scale-up” roadmap represents the most ambitious computing infrastructure plan in history. The scale of computation being planned will fundamentally transform AI economics. AI workloads currently reserved for high-value business processes (such as the recently launched OpenAI o1-Pro API priced at a staggering $600 per million output tokens, 1,000x more than Gemini 2.0 Flash) becomes accessible for countless everyday applications. Complex reasoning that once required prohibitive amounts of computing will become standard. This efficiency revolution explains why Nvidia's vision isn't at odds with models like DeepSeek that use less computing power. Both trends, more efficient models and more powerful hardware, work together to expand the AI market. As the markets look less favourably on Nvidia it’s clear that unlike ExoBrain, few market participants have spent much time building agents and sensing the tantalising opportunities that await with future GPU economics.
Adobe orchestrates autonomous marketing
At this week's Adobe Summit in Las Vegas, the company unveiled its next major AI push: 10 new AI agents and an "agent orchestrator" system. This marks Adobe's entry into what they call the third phase of AI evolution - agentic AI - following descriptive and generative phases. Unlike Salesforce, which offers the Agentforce 2dx platform for building agents, Adobe's approach seems more pre-packaged: 10 specific agents aimed at solving real marketing problems. These include audience targeting, data analysis, and site optimisation tools.
Adobe is positioning itself as the central "orchestrator" of marketing AI, announcing partnerships with Microsoft, AWS, and agency groups like Publicis and WPP. The agents aim to deliver on Adobe's vision of "personalisation at scale" - helping brands create highly targeted content efficiently. This push will naturally raise questions about the future of marketing roles. While Adobe frames these tools as "copilots" that free humans for strategic thinking, the reality of automated marketing workflows suggests potential disruption for various roles and business models.
Takeaways: With the likes of Adobe and Zoom making big announcements, there’s no question that agentic AI is the buzz concept for enterprise software in 2025. These tools will likely reshape marketing teams while offering new ways to construct highly adaptable and reactive communication flows. The test in the short term will be whether the execution can align with the hype, and we see a real shift into digital labour driven productivity.
Agent capability is doubling every 7 months

AI systems' ability to handle complex tasks is growing at a rapid rate - doubling every seven months according to new METR research. The chart shows progression from GPT-2's basic capabilities to today's models tackling hour-long tasks. If this trend continues, by 2027 could AI manage full eight-hour workday? Even with imperfect accuracy (50%), this transforms economics when verification costs remain low. The question isn't if AI will handle longer tasks, but how we'll adapt our workflows around different reliability thresholds and build systems that combine AI speed with human oversight. At ExoBrain we use Devin, the autonomous (agent) engineer. It can work to up to 30 minutes on a complex task with reasonable results although things drop-off if the work gets more difficult. What we’re seeing and what this research suggests however is that there may be a new Moore's Law for agent value. The race is on to unlock hybrid workflows that combine agent endurance with human quality control and coordination.
Weekly news roundup
This week saw major developments in AI infrastructure and hardware investments, continued evolution of AI governance challenges, and significant breakthroughs in research efficiency and model development.
AI business news
Perplexity's bid for TikTok continues (Shows how AI companies are looking to expand into social media and content discovery)
OpenAI upgrades its transcription and voice-generating AI models (Demonstrates continued advances in AI audio capabilities that could transform content creation)
British start-up wins £1 million AI prize for breakthrough slashing materials development from years to days (Highlights AI's potential to accelerate scientific discovery)
Deloitte hops aboard the agentic AI train with Zora AI (Shows growing enterprise adoption of autonomous AI agents)
Nvidia bets big on synthetic data (Indicates the growing importance of synthetic data in AI training)
AI governance news
Trapping misbehaving bots in an AI labyrinth (Showcases innovative approaches to AI security)
Andor creator refuses to publish scripts thanks to AI (Illustrates growing creative industry concerns about AI training)
ChatGPT falsely told man he killed his children (Highlights ongoing challenges with AI hallucinations)
Court rules copyrighting AI-generated art is a no-go - even if you invented the software (Sets important precedent for AI copyright law)
Report co-authored by Fei-Fei Li stresses need for AI regulations to consider future risks (Shows leading experts pushing for forward-looking AI regulation)
AI research news
DAPO: An open-source LLM reinforcement learning system at scale (Provides new tools for improving LLM training)
Why we can't escape brute-force search (Explains fundamental limitations in AI development)
Survey on evaluation of LLM-based agents (Helps standardise how we assess AI agent capabilities)
SmolDocling: An ultra-compact vision-language model for end-to-end multi-modal document conversion (Advances efficient document processing)
Charting and navigating Hugging Face's model atlas (Makes AI model selection more accessible)
AI hardware news
Microsoft had $12 billion Coreweave option, deferred to OpenAI (Shows strategic AI infrastructure decisions at tech giants)
Synopsys lays out strategy for AI 'agents' to design computer chips (Demonstrates AI's role in chip design automation)
SoftBank buys server-grade Arm silicon designer Ampere (Indicates growing investment in AI chip development)
Nvidia debuts new silicon photonics switches for AI data centers (Shows advances in AI data center infrastructure)
AI cloud operator CoreWeave files for $2.7B IPO (Reflects growing market for specialised AI infrastructure)